class: center, middle, inverse, title-slide # The war against p values in medical research ### Claus Thorn Ekstrøm and Theis Lange
UCPH Biostatistics
.small[
ekstrom@sund.ku.dk
] / .small[
thlan@sund.ku.dk
]
@ClausEkstrom
/
@LangeTheis
### YSD Jan 16th, 2020
.small[Slides:
biostatistics.dk/talks/
] --- class: middle, center, inverse # Quiz --- # What is a `\(p\)` value anyway? > *The `\(p\)` value is the probability of having obtained a result **at least as extreme** as the one found with our sample if the null hypothesis were true.* --- Kirkwood & Sterne -- IF the null hypothesis is true<br> .yellow[AND all the other assumptions about the model are *also* true]<br> THEN the `\(p\)` value expresses the probability of observing something as least as extreme as what you have in your sample. ??? If A is TRUE then B cannot occur; However, B has occurred; Therefore A is false If A is TRUE then B probably cannot occur; However, B has occurred; Therefore A is probably false --- # What is a `\(p\)` value anyway? > *The `\(p\)` value is the probability of having obtained a result **at least as extreme** as the one found with our sample if the null hypothesis were true.* --- Kirkwood & Sterne IF the null hypothesis is true<br> .yellow[AND all the other assumptions about the model are *also* true]<br> THEN the `\(p\)` value expresses the probability of observing something as least as extreme as what you have in your sample. Roughly: the `\(p\)` value is a number that measures how surprised you are. --- # The epistemology of science .center[ <img src="pics/process.png" width="500" /> ] --- background-image: url(pics/indded.png) background-size: 100% --- class: inverse, middle, center # A history of the (war against) p values --- # From ancient times ... <img src="pvalues_files/figure-html/unnamed-chunk-2-1.png" width="100%" /> ??? Popper did not like the probability argument --- # ... to recent times 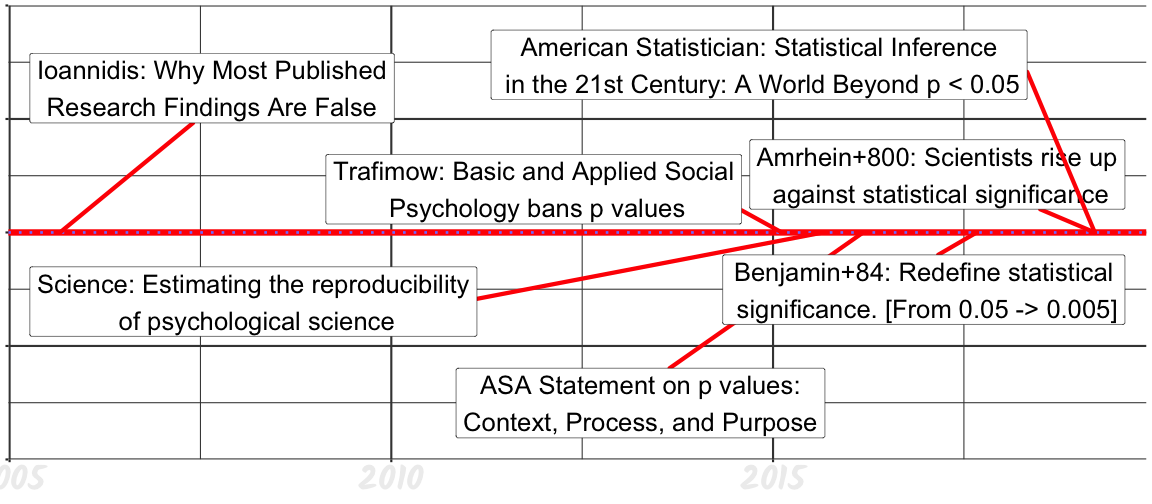<!-- --> --- # What are the problems with p values? They try to answer the *"wrong"* question A researcher typically wants to know if the hypothesis holds: `$$P(H | D)$$` but the p value computes `$$P(D \text{ "or more extreme" } | H)$$` ??? They give a very precise answer to the wrong question instead of an approxiomate answer to the right question. --- # The p value is used in the wrong way Typically used as a decision rule: `$$p \text{ value} \left\{\begin{array}{ll}<0.05 & \text{reject} - \text{"significant"} \\ \geq 0.05 & \text{not reject} - \text{"not significant" or "no association"} \end{array} \right.$$` * Arbitrary threshold for continuous scale * Significant does not mean clinically relevant * Non-significance does not mean that `\(H_0\)` is true - only that there was insufficient evidence to reject it (*"absence of evidence is not evidence of absence"*). ??? "No association" is wrong to say binary thinking makes everything worse in that people inappropriately combine probabilistic statements with Boolean rules. --- # The p value contains two types of information .pull-left[The p value combines information about the *effect size* and *sample size*. When `\(N\rightarrow\infty\)` *everything* becomes significant. ] .pull-right[ <img src="pics/donkey.jpg" width="100%" /> ] --- # Unrealistic null hypothesis Compare two treatments with effects `\(\mu_1\)` and `\(\mu_2\)` `$$H_0 : \mu_1 = \mu_2$$` When do we really believe that the effects of two treatments are *exactly* the same? Hard to believe in most public health or social science research. ??? At least outside randomization --- background-image: url(pics/theis1.png) background-size: 100% --- background-image: url(pics/theis2.png) background-size: 100% --- class: inverse, middle, center # Alternative proposals --- # Use confidence intervals The CI is defined as the values of `\(H_0\)` that are *not* rejected. 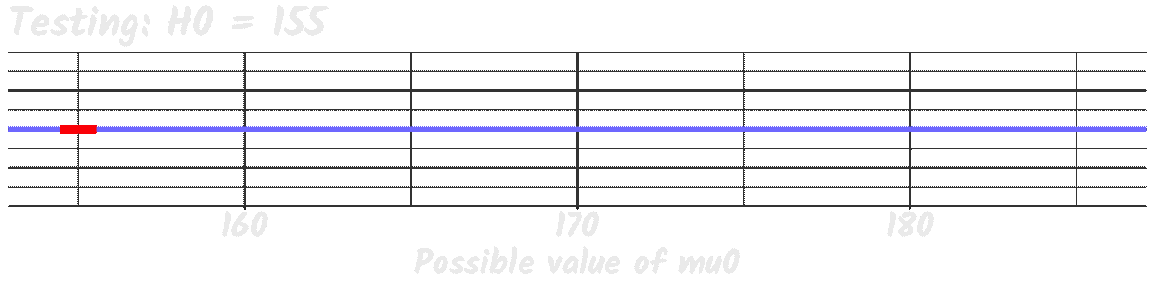<!-- --> Fully defined from (infinitely many) p values --- # ... interpretation of the CI .pull-left[ <img src="pvalues_files/figure-html/unnamed-chunk-6-1.png" width="100%" /> <img src="pvalues_files/figure-html/unnamed-chunk-7-1.png" width="100%" /> ] -- .pull-right[ **Epidemiologists:** interpret confidence intervals as credible intervals. **Biostatisticians:** Know that CIs are not credible intervals, but interpret them as though they were anyway. ] --- # Bayes factors .pull-left[ The Bayes factor is the ratio of the likelihood of two hypotheses: `$$BF = \frac{P(D | H_1)}{P(D | H_0)}$$` Move problem to another scale! **Several** other problems. ] .pull-right[ <img src="pvalues_files/figure-html/unnamed-chunk-8-1.png" width="100%" /> ] ??? If you think p values are problematic, wait until you understand Bayes facts depend crucially on aspects of the prior distribution that are typically assigned in a completely arbitrary manner by users. IF B10 IS… THEN YOU HAVE… > 100 Extreme evidence for H1 30 – 100 Very strong evidence for H1 10 – 30 Strong evidence for H1 3 – 10 Moderate evidence for H1 1 – 3 Anecdotal evidence for H1 1 No evidence 1/3 – 1 Anecdotal evidence for H1 1/3 – 1/10 Moderate evidence for H1 1/10 – 1/30 Strong evidence for H1 1/30 – 1/100 Very strong evidence for H1 < 1/100 Extreme evidence for H1 --- # Lower the significance level .pull-left[ .small[ Pros: * Fewer false positives * Improve replicability Cons: * More false negatives * Still dichotomizes results * Does not fix **any** of the conceptual problems with the p value ] ] .pull-right[ Use `\(\alpha\)`=0.005 instead of 0.05. <img src="pics/redefine.png" width="100%" /> ] --- # Bayesian analysis .pull-left[ Answers the "right" question: *What is the probability that my hypothesis holds?* `$$P(H|D)$$` * Subjective vs objective * Moves discussion to priors ] .pull-right[ Posterior distribution of `\(\theta\)` 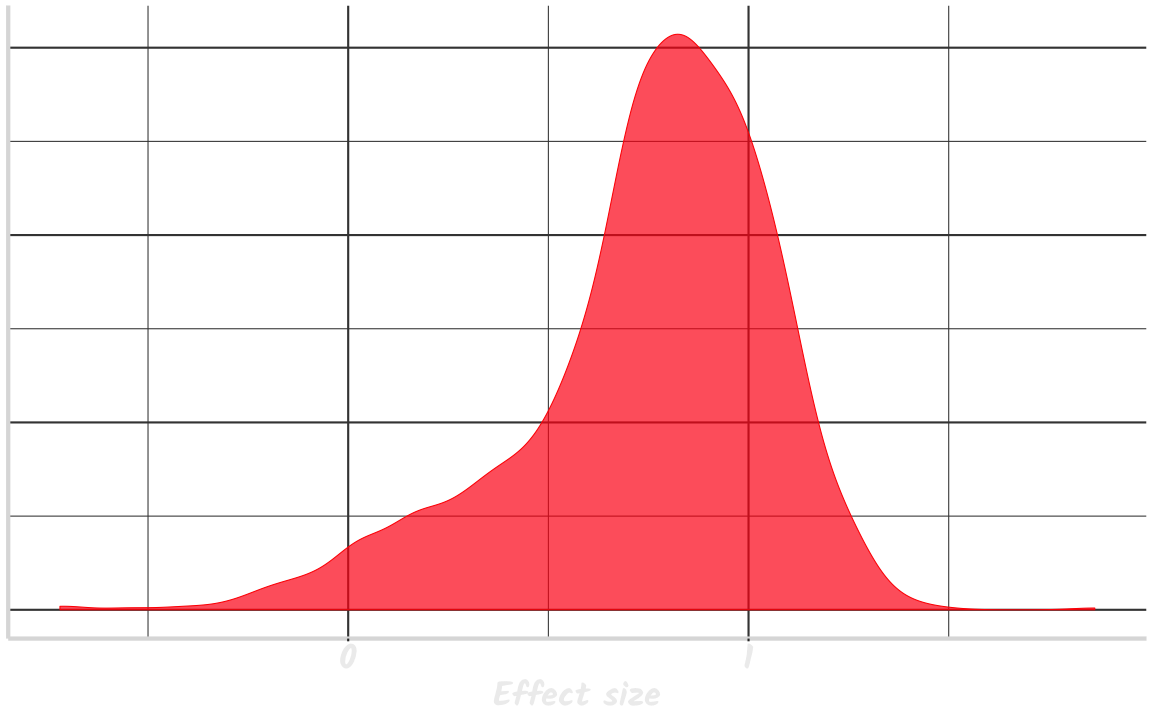<!-- --> ] --- background-image: url(pics/theis3.png) background-size: 100% --- background-image: url(pics/iceberg.jpg) background-size: 100% # Let's put things into perspective -- .pull-left[ * Which variables? * How to measure? * Missing data * Entry errors * Which model? * Which specification? * Which assumptions? ] .pull-right[ * Confounding * Collinearity * Overfitting * `\(p\)` hacking * Interpretation * Published? * Replicated? ] --- # Publication bias .footnotesize[ .pull-left6[ | Scientist | Salesman | |:-----------|:-------------| | Be sceptical of your results | "Sell" your results | | Interpret conclusions carefully | Highlight / exaggerate importance | | "Publish" negative results | Publish strategically | | Replicate replicate replicate | Replicate ... if you must | | Novel exciting results are less likely to be true | Publish novel results before they get scooped | ] ] .pull-right6[ <img src="pics/elephant.jpg" width="100%" /> ] --- class: inverse, middle, center # What about the future? --- # Are p values bad? * No -- * Medicine / public health has moved forward in leaps and bounds in the last 100 years. -- * *"Assessing the Statistical Analyses Used in *Basic and Applied Social Psychology* After Their p-Value Ban" (2019)* -- * The function of significance tests is to *prevent you from making a fool of yourself*, and not to make unpublishable results publishable ??? If a drunken driver crashes into a tree it is not the cars fault (at least not yet). 31 BASP papers. 17 with some statistics --- # Recommendations Embrace uncertainty! Know your tools! * Report effect sizes and CIs (and perhaps p values) * Put as much energy into discussing clinical relevance as statistical results. * Abandon dichotomizing and "statistically significant" * Never conclude: "no difference" eller "no effect" ??? Present statistical conclusions with uncertainty rather than as dichotomies March 2019, 800 scientists har skrevet under. 1: never conclude: no difference eller no association 2: abandon dichotomizing and "statistically significant" --- # Who said this? > *Some people hate the very name of statistics, but I find them full of beauty and interest. Whenever **they are not brutalized**, but **delicately handled** by the higher methods, and are **warily interpreted**, their power of dealing with complicated phenomena is extraordinary. They are the **only tools** by which an opening can be cut through the formidable thicket of difficulties that bars the path of those who pursue the Science of man.* -- > *- Francis Galton (1894)* ??? We can provide the best methods possible. it is up to the researcher to apply them (delicately handled) and appropirately decipher the results "warily interpreted" --- # References .small[ * Amrhein V, Greenland S & McShane B (2019) [Scientists rise up against statistical significance](https://www.nature.com/articles/d41586-019-00857-9) * Benjamin et al (2018) [Redefine statistical significance](https://www.nature.com/articles/s41562-017-0189-z). Nature Human Behaviour. * Fisher, R. (1925). [Statistical Methods for Research Workers](http://psychclassics.yorku.ca/Fisher/Methods/) * Fisher, R. (1935). [The Logic of Inductive Inference](https://www.jstor.org/stable/2342435?seq=1#metadata_info_tab_contents), JRSS * Fricker et al. (2019). [Assessing the Statistical Analyses Used in *Basic and Applied Social Psychology* After Their p-Value Ban](https://www.tandfonline.com/doi/full/10.1080/00031305.2018.1537892) * Held, L (2019). [A New Standard for the Analysis and Design of Replication Studies](https://arxiv.org/pdf/1811.10287.pdf) * Ioannidis, J. (2005) [Why Most Published Research Findings Are False](https://journals.plos.org/plosmedicine/article?id=10.1371/journal.pmed.0020124) PLoS Medicine ] --- .small[ * Lindquist, EF (1940). Statistical analysis in educational research. * Mayo, D. (2018): [Statistical Inference as Severe Testing : How to Get Beyond the Statistics Wars](https://amzn.to/2Q5rAiJ) * Neyman J., Pearson E. S. (1928). [On the use and interpretation of certain test criteria for purposes of statistical inference: part I.](https://www.jstor.org/stable/2331945?seq=1#metadata_info_tab_contents) Biometrika 20A, 175–240. * Popper K (1934) [Logik der Forschung](http://strangebeautiful.com/other-texts/popper-logic-scientific-discovery.pdf) * Sheldon, N. (2019), [What does it all mean?](https://rss.onlinelibrary.wiley.com/doi/10.1111/j.1740-9713.2019.01296.x) Significance, 16: 15-17. * Trafimow, D. & Marks, M. (2015) [Editorial](https://www.tandfonline.com/doi/full/10.1080/01973533.2015.1012991), Basic and Applied Social Psychology, 37, 1-2. * Wasserstein, R.L. & Lazar, N.A. (2016) [The ASA Statement on p-Values: Context, Process, and Purpose](https://amstat.tandfonline.com/doi/full/10.1080/00031305.2016.1154108), The American Statistician, 70:2, 129-133. * Open Science Collaboration (2015), [Estimating the reproducibility of psychological science](https://science.sciencemag.org/content/349/6251/aac4716). Science. ]